State of AI Agents
We surveyed over 1,300 professionals — from engineers and product managers to business leaders and executives — to uncover the state of AI agents. Dive into the data as we break down how AI agents are being used (or not) today.
Introduction
In 2024, AI agents are no longer a niche interest. Companies across industries are getting more serious about incorporating agents into their workflows - from automating mundane tasks, to assisting with data analysis or writing code.
But what’s really happening behind the scenes? Are AI agents living up to their potential, or are they just another buzzword? Who’s been deploying them, and what’s preventing others from diving in headfirst?
We surveyed over 1,300 professionals to learn about the state of AI agents in 2024. Let's dive into the data below.
Insights
First, what even is an agent?
At LangChain, we define an agent as a system that uses an LLM to decide the control flow of an application. Just like the levels of autonomy for autonomous vehicles, there is also a spectrum of agentic capabilities.
Agent adoption is a coin toss - but nearly everyone has plans for it
The agent race is heating up. In the past year, numerous agentic frameworks have gained enormous popularity — whether it’s using ReAct to combine LLM reasoning and acting, multi-agent orchestrators, or a more controllable framework like LangGraph.
Not all of the chatter on agents is Twitter hype. About 51% of respondents are using agents in production today. When we looked at the data by company size, mid-sized companies (100 - 2000 employees) were the most aggressive with putting agents in production (at 63%).
Encouragingly, 78% have active plans to implement agents into production soon. While it’s clear that the appetite for AI agents is strong, the actual production deployment still remains a hurdle to many.
agents in production?
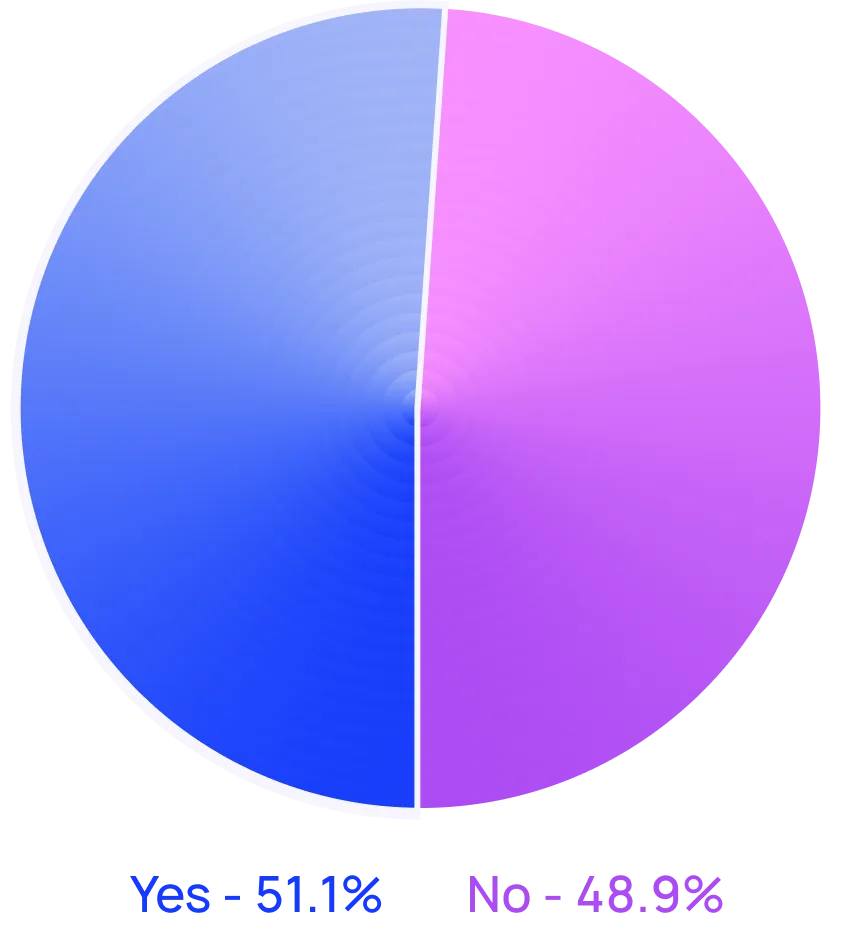
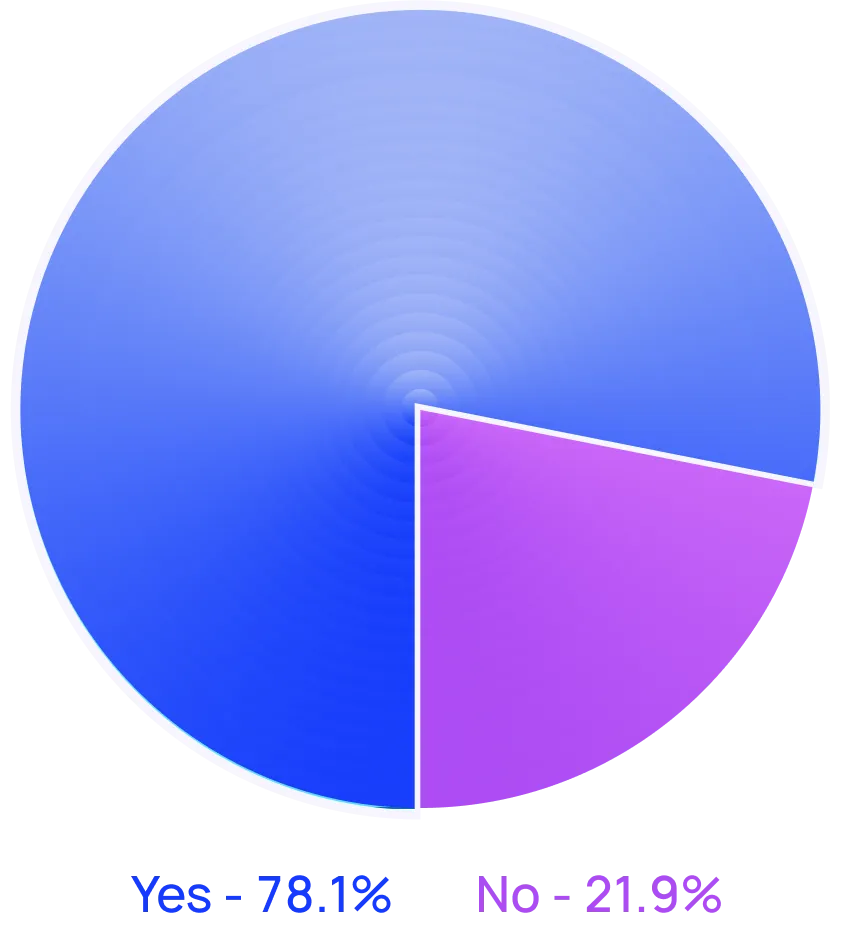
We also continue to see companies moving beyond simple chat-based implementations into more advanced frameworks that emphasize multi-agent collaboration and more autonomous capabilities. (See more in the "Emerging themes" section below.)
While the tech industry is usually known to be early adopters, interest in agents is gaining traction across all industries. 90% of respondents working in non-tech companies have or are planning to put agents in production (nearly equivalent to tech companies, at 89%).
Leading agent use cases
What are people using agents for? Agents are handling both routine tasks but also opening doors to new possibilities for knowledge work.
The top use cases for agents include performing research and summarization (58%), followed by streamlining tasks for personal productivity or assistance (53.5%).
These speak to the desire of people to have someone (or something) else handle time-consuming tasks for them. Instead of sifting through endless data for literature review or research analysis, users can rely on AI agents to distill key insights from volumes of information. Similarly, AI agents are boosting personal productivity by assisting with everyday tasks like scheduling and organization, freeing users to focus on what matters.
Efficiency gains aren’t limited to the individual. Customer service (45.8%) is another prime area for agent use cases, helping companies handle inquiries, troubleshoot, and speed up customer response times across teams.
Better safe than sorry: Tracing and human oversight are needed to keep agents in check
With great power comes great responsibility — or at least the need for some brakes and controls for your agent. Tracing and observability tools top the list of must-have controls, helping developers get visibility into agent behaviors and performance. Most companies are also employing guardrails to keep agents from veering off course.

When it came to testing LLM applications, offline evaluation (39.8%) was mentioned as a strategy more often than online evaluation (32.5%). This may speak to the difficulty of monitoring real-time performance. In the write-in responses, many companies also had human experts manually checking or evaluating responses for an added layer of precaution.
Despite the excitement that folks have projected onto agents, most are taking a more conservative approach when it comes to how far we’ll let agents go off the leash. Very few respondents allow their agent to read, write, and delete freely. Instead, most teams allow either read-only tool permissions or require human approval for more significant actions, such as writing or deleting.
Companies of different sizes also weight their priorities differently when it comes to agent controls. Unsurprisingly, larger enterprises (2000+ employees) are more cautious, leaning heavily on “read-only” permissions to avoid unnecessary risks. They also tend to pair guardrails with offline evaluations to catch regressions in pre-production, before customers see any responses.
Meanwhile, small companies and startups (<100 employees) are more focused on tracing to understand what’s happening in their agentic app (over other controls). From our conversations, smaller companies tend to focus on shipping and understanding the results by just looking at the data; whereas enterprises put more controls in place across the board.
While rates of agent adoption were similar across non-tech and tech company respondents, among those using agent controls in production, tech companies were more likely to use multiple control methods. 51% of tech respondents are currently using 2 or more control methods, compared to only 39% of respondents in other sectors. This suggests that tech companies may be further along in building reliable agents, as controls are needed for high-quality experiences.
Agent success stories: Cursor steals the spotlight
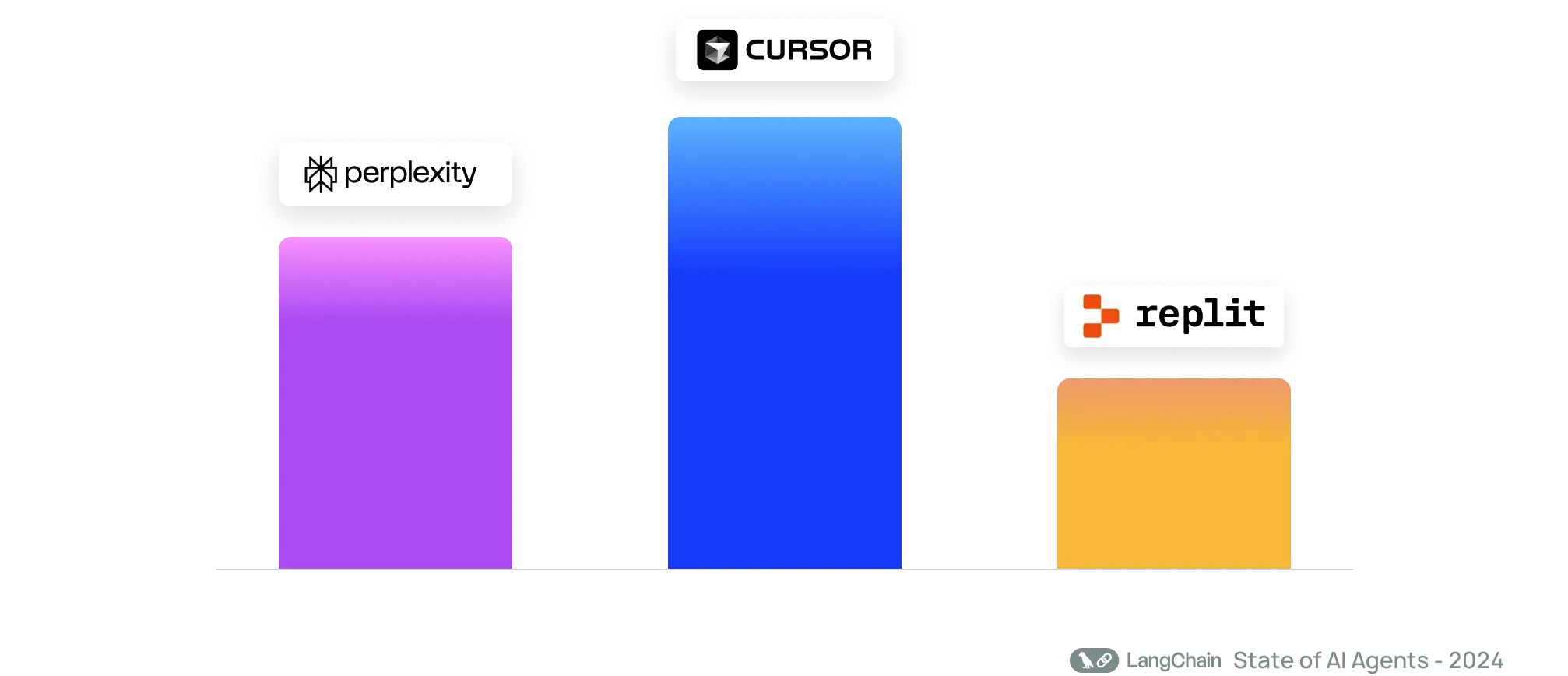
Cursor takes the crown as the most talked-about agent application in our survey, followed closely by heavyweights like Perplexity and Replit.
Cursor is an AI-powered code editor that helps developers write, debug, and parse code with smart autocompletes and contextual assistance. Replit also accelerates the software development lifecycle by setting up environments, configurations, and letting you build and deploy fully functional apps in minutes. Perplexity is an AI-powered answer engine that can answer complex queries with web search and link sources in its responses.
These applications are pushing the boundaries of what agents can do, showing that AI agents are no longer theoretical — they’re solving real problems in production environments today.
Emerging themes in AI agent adoption
From our write-in responses, we see a number of evolving expectations and challenges organizations face as they bring AI agents into their workflows.
There is an admiration for these capabilities of AI agents:
But there are also challenges to consider for teams building agents. This includes:
• Barriers to understanding agent behavior. Several engineers wrote in about their difficulties in explaining the capabilities and behaviors of AI agents to other stakeholders in their companies. Sometimes a little extra visualization of steps can explain what happened with an agent response. Other times, the LLM is still a blackbox. The additional burden of explainability is left with the engineering team.
In spite of the challenges, there is notable buzz and energy around the following areas:
Conclusion
The race to integrate AI agents is on, as companies are already starting to reshape workflows and design their future with LLMs at the helm of improved decision making and human productivity.
But while excitement is high, companies are also aware that they must tread carefully, seeding the right controls to navigate new use cases and applications. Teams are eager but cautious, experimenting with frameworks to try to keep their agent responses high-quality and hallucination-free.
As we look ahead, companies who can crack the code on reliable, controllable agents will have a headstart in the next wave of AI innovation - and begin setting the standard for the future of intelligent automation.
Methodology
Top 5 industries:
- Technology (60% of respondents)
- Financial Services (11% of respondents)
- Healthcare (6% of respondents)
- Education (5% of respondents)
- Consumer Goods (4%)
Company size:
- <100 people (51% of respondents)
- 100-2000 people (22% of respondents)
- 2000-10,000 people (11% of respondents)
- 10,000+ people (16% of respondents)